Zhang, Jinwei, Zuo, Lianrui, Dewey, Blake E., Remedios, Samuel W., Pham, Dzung L., Carass, Aaron, & Prince, Jerry L. (2024). Towards an accurate and generalizable multiple sclerosis lesion segmentation model using self-ensembled lesion fusion. In Proceedings of the 21st IEEE International Symposium on Biomedical Imaging (ISBI 2024), Athens, Greece, May 27-30, 2024. https://doi.org/10.1109/ISBI56570.2024.10635877
This study focuses on improving the automatic detection and segmentation of multiple sclerosis (MS) lesions in MRI scans, a process that is crucial for efficient and consistent diagnosis but traditionally performed manually. Current automated methods often rely on complex modifications to U-Net-based architectures to improve performance. However, these modifications can limit how well the models generalize across different MRI datasets with varying contrasts or image quality.
The researchers aimed to address this by developing a segmentation model based on the standard U-Net architecture, without any additional modifications, to create a more accurate and generalizable tool for detecting MS lesions. They introduced a novel self-ensembling technique that enhances model performance during testing by combining multiple predictions into a final, refined segmentation. This approach not only achieved the highest performance in the widely recognized ISBI 2015 MS lesion segmentation challenge but also showed that it is robust across different settings of the self-ensemble parameters.
Additionally, the study found that using instance normalization, rather than the more common batch normalization, improved the model’s ability to generalize across clinical MRI data from various scanners, making it more versatile in real-world applications. This work demonstrates that sophisticated modifications to architectures are not always necessary to achieve high accuracy and that simple techniques, when applied thoughtfully, can result in highly generalizable and efficient models for MS lesion segmentation.
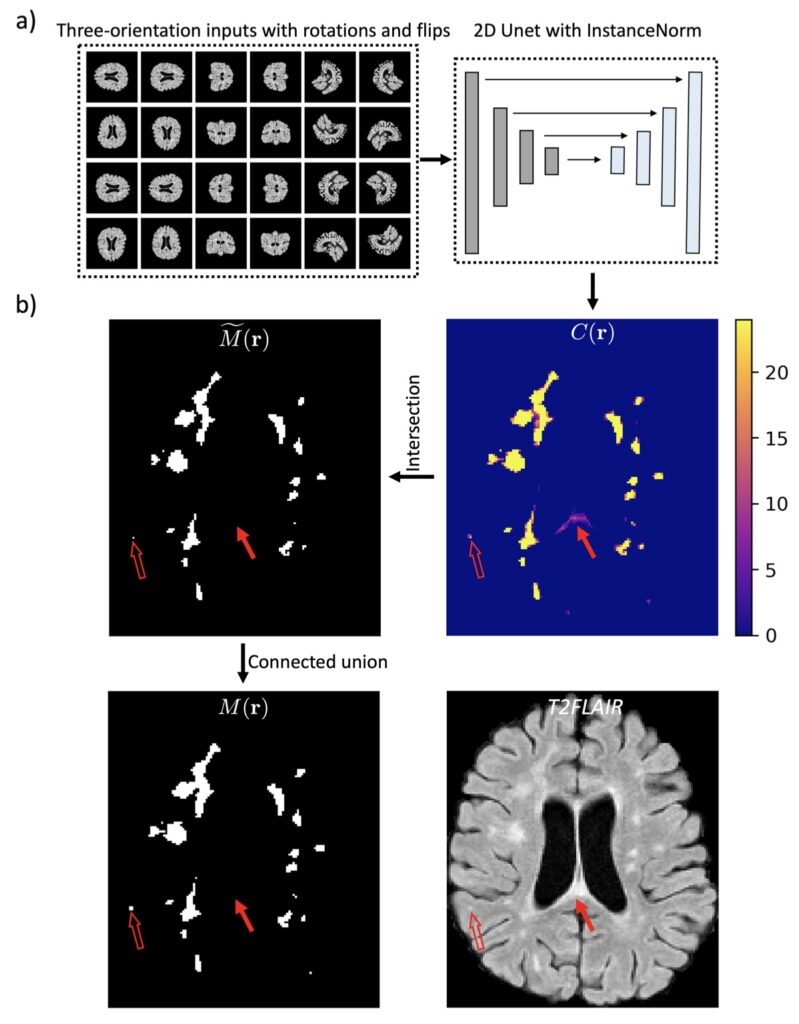