Bao, Shunxing, Guo, Junlin, Lee, Ho Hin, Deng, Ruining, Cui, Can, Remedios, Lucas W., Liu, Quan, Yang, Qi, Xu, Kaiwen, Yu, Xin, Li, Jia, & Li, Yike. (2024). Mitigating over-saturated fluorescence images through a semi-supervised generative adversarial network. In Proceedings of the 21st IEEE International Symposium on Biomedical Imaging (ISBI 2024), Athens, Greece, May 27-30, 2024. https://doi.org/10.1109/ISBI56570.2024.10635687
This study addresses a key challenge in multiplex immunofluorescence (MxIF) imaging, a technique used in biomedical research to provide detailed insights into cell structures and spatial organization. While MxIF imaging, such as using DAPI staining to identify cell nuclei and CD20 staining for cell membranes, is invaluable for understanding cell composition, it suffers from saturation artifacts. These artifacts occur when certain areas of the image become overly bright, making it difficult to analyze individual cells accurately. Existing methods for correcting these saturation issues, like gamma correction, often fall short because they assume uniform saturation, which is rarely the case in practice.
The authors propose a novel solution using a hybrid generative adversarial network (GAN) called HD-mixGAN, which combines two different types of neural networks (CycleGAN and Pix2pixHD) to correct saturation artifacts. This approach takes advantage of both small datasets where paired (before and after) images are available and larger datasets that only have unpaired images of over-saturated regions. By generating synthetic data from the unpaired datasets using a CycleGAN and combining it with real data, the model effectively learns to correct saturation artifacts, improving the overall image quality.
The method was tested in a task to detect cell nuclei, where it significantly outperformed traditional methods, improving the accuracy (F1 score) by 6%. This approach represents the first focused effort to address saturation issues in multi-round MxIF imaging, providing a data-driven solution that enhances the accuracy of single-cell analysis. The study also makes its code and implementation freely available, facilitating further research and applications in this area.
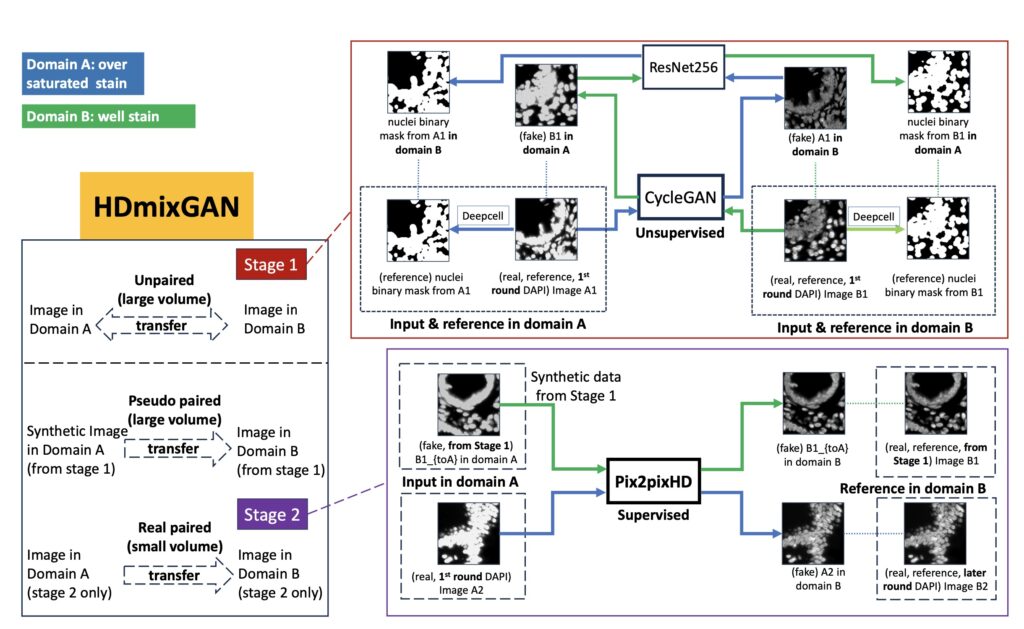
The authors propose a novel solution using a hybrid generative adversarial network (GAN) called HD-mixGAN, which combines two different types of neural networks (CycleGAN and Pix2pixHD) to correct saturation artifacts. This approach takes advantage of both small datasets where paired (before and after) images are available and larger datasets that only have unpaired images of over-saturated regions. By generating synthetic data from the unpaired datasets using a CycleGAN and combining it with real data, the model effectively learns to correct saturation artifacts, improving the overall image quality.
The method was tested in a task to detect cell nuclei, where it significantly outperformed traditional methods, improving the accuracy (F1 score) by 6%. This approach represents the first focused effort to address saturation issues in multi-round MxIF imaging, providing a data-driven solution that enhances the accuracy of single-cell analysis. The study also makes its code and implementation freely available, facilitating further research and applications in this area.