Hoang, Nhung, Sardaripour, Neda, Ramey, Grace D., Schilling, Kurt, Liao, Emily, Chen, Yiting, Park, Jee Hyun, Bledsoe, Xavier, Landman, Bennett A., Gamazon, Eric R., Benton, Mary Lauren, & Capra, John A., Rubinov, Mikail. (2024). Integration of estimated regional gene expression with neuroimaging and clinical phenotypes at biobank scale. PLoS Biology, 22(9), e3002782. https://doi.org/10.1371/journal.pbio.3002782
This study aims to deepen our understanding of human brain individuality by integrating various large-scale data sets, including genomic, transcriptomic, neuroimaging, and electronic health records. The researchers used computational genomics methods to estimate genetically regulated gene expression (gr-expression) for 18,647 genes across 10 brain regions in over 45,000 people from the UK Biobank. Their analysis revealed that gr-expression patterns align with known genetic ancestry relationships, brain region identities, and gene expression correlations across different regions.
Through transcriptome-wide association studies (TWAS), they discovered 1,065 associations between gr-expression and individual differences in gray matter volumes across people and brain regions. These findings were compared to genome-wide association studies (GWAS) in the same sample, revealing hundreds of novel associations. The study also linked gr-expression to clinical phenotypes by integrating results from the Vanderbilt Biobank.
Further analysis involved the Human Connectome Project (HCP), where they identified associations between polygenic gr-expression and MRI-based structural and functional brain phenotypes. The results were highly replicable, strengthening the reliability of their findings. Overall, this work offers a valuable new resource for connecting genetically regulated gene expression to brain organization and diseases, advancing our understanding of brain individuality and its clinical relevance.
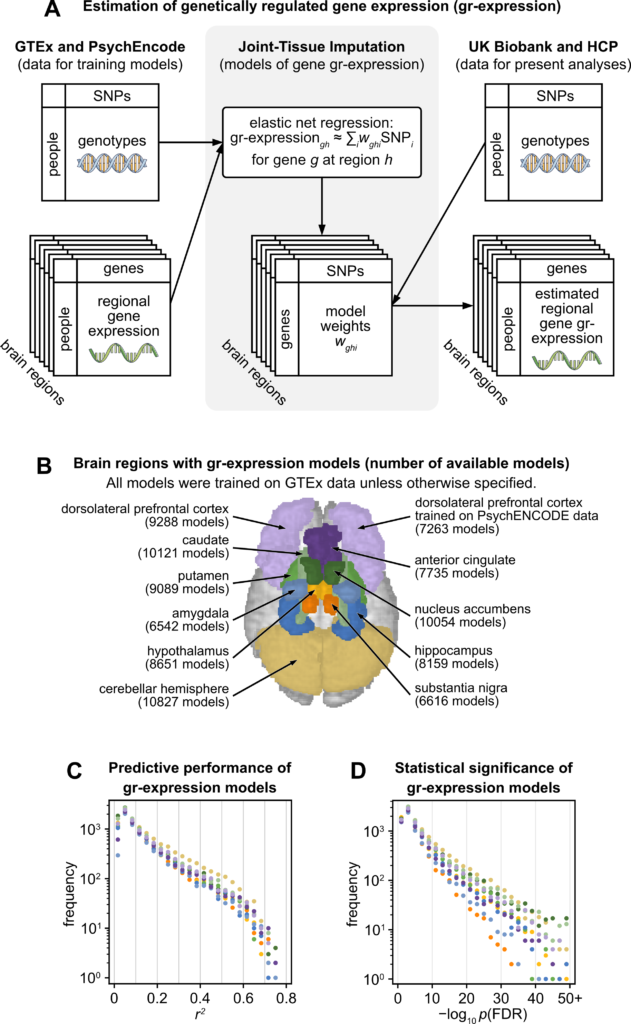
(A) Pipeline for estimation of gr-expression with Joint-Tissue Imputation. Left: Joint-Tissue Imputation models are trained on genetic sequences and directly assayed gene expression from postmortem brain samples in the GTEx and PsychEncode projects. Center: The models are trained to estimate gr-expression as a weighted sum of SNPs that are close to the gene of interest along the linear genome. The estimation includes elastic-net regularization because the number of these SNPs typically exceeds the number of samples in the training data. Right: The trained models were used to estimate gr-expression from genetic sequences of neuroimaging-genomic samples in the UK Biobank and the HCP. (B) An illustration of the 10 cortical and subcortical regions with available models of gr-expression. Numbers in parentheses refer to all models that passed baseline performance thresholds for the prediction of observed gene expression on held-out data (r2 > 0.01 and pFDR < 0.05). (C, D) Predictive performance of gr-expression models on held-out data from the GTEx data set. (C) Histograms of r [2], the variance of directly assayed gene expression explained by estimated gr-expression. (D) Histograms of p-values (−log10 pFDR) on these r2 values. Regions are colored as in panel B. FDR, false discovery rate; GTEx, Genotype-Tissue Expression Project; HCP, Human Connectome Project; SNP, single-nucleotide polymorphism.