Gao, Chenyu, Bao, Shunxing, Kim, Michael E., Newlin, Nancy R., Kanakaraj, Praitayini, Yao, Tianyuan, Rudravaram, Gaurav, Huo, Yuankai, Moyer, Daniel, Schilling, Kurt, Kukull, Walter A., & Toga, Arthur W. (2024). Field-of-view extension for brain diffusion MRI via deep generative models. Journal of Medical Imaging, 11(4), 044008. https://doi.org/10.1117/1.JMI.11.4.044008
This study focuses on improving brain diffusion magnetic resonance imaging (dMRI) when the field of view (FOV) is incomplete, a common issue that can hinder analysis of brain tissue microstructure and connectivity. Rather than discarding corrupted dMRI data due to missing slices, the authors propose a deep generative model to impute, or estimate, the absent brain regions directly from existing scans. This allows for more complete whole-brain tractography, which is essential for mapping brain connections.
The framework leverages both the diffusion characteristics from diffusion-weighted images (DWIs) and anatomical details from structural images to accurately reconstruct missing slices in the dMRI data. Testing on the Wisconsin Registry for Alzheimer’s Prevention (WRAP) and National Alzheimer’s Coordinating Center (NACC) datasets showed that the imputed slices significantly improved the accuracy of whole-brain tractography. Specifically, the model enhanced the precision of tractography for 72 brain tracts, resulting in improved analysis of brain connectivity, which is crucial for studying diseases like Alzheimer’s.
Overall, this approach provides an effective alternative to discarding incomplete dMRI data, enabling more reliable analyses by reconstructing missing information and extending the FOV. The method helps reduce uncertainty in tractography, offering valuable insights into brain structure, especially in clinical studies related to neurodegenerative conditions.
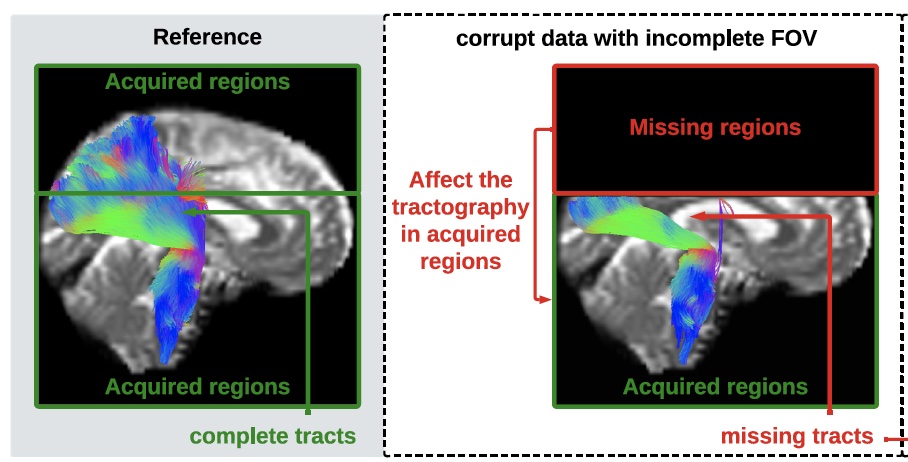
impossible but can also impact the tractography performed in the acquired regions [as shown in
panel (b)], e.g., yielding missing streamlines of corticospinal tract (CST) compared with the reference
[as shown in panel (a)].