Zhang, Liting; Zhou, Xin Maizie; Mallory, Xian. “SCCNAInfer: a robust and accurate tool to infer the absolute copy number on scDNA-seq data.” Bioinformatics, Volume 40, Issue 7, July 2024, btae454, https://doi.org/10.1093/bioinformatics/btae454.
In diseases like cancer, changes in our cells called copy number alterations (CNAs) are important to understand. These changes can tell us a lot about how diseases progress. Single-cell DNA sequencing (scDNA-seq) helps researchers detect CNAs in individual cells, but current tools can make mistakes across the entire genome due to wrong estimates of cell chromosome numbers, or “ploidy.”
SCCNAInfer is a new tool designed to improve this process. It uses information from inside tumor cells to more accurately estimate each cell’s ploidy and CNAs. SCCNAInfer works alongside existing CNA detection methods by grouping cells, calculating ploidy for each group, refining the data, and accurately identifying CNAs for each cell.
Tests show that SCCNAInfer does a better job compared to other tools like Aneufinder, Ginkgo, SCOPE, and SeCNV. This new tool can help researchers get clearer insights into cell changes, aiding in the study of cancer and other diseases.
SCCNAInfer is freely available at https://github.com/compbio-mallory/SCCNAInfer.
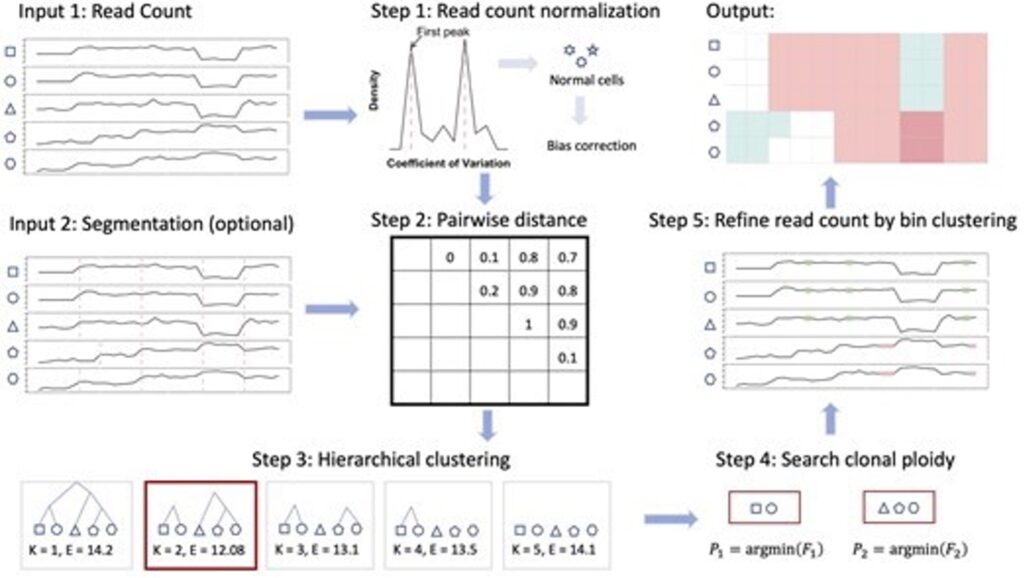