Hu, Yunfei; Xie, Manfei; Li, Yikang; Rao, Mingxing; Shen, Wenjun; Luo, Can; Qin, Haoran; Baek, Jihoon; Zhou, Xin Maizie. “Benchmarking clustering, alignment, and integration methods for spatial transcriptomics.” Genome Biology, volume 25, Article number: 212 (2024). https://doi.org/10.1186/s13059-024-02665-w. Published: 09 August 2024.
Understanding the complexities of tissues and organisms is no small feat. However, scientists are making great strides with a cutting-edge technique called spatial transcriptomics (ST). This method allows us to study tissues at a microscopic level, revealing valuable information about their structure and function.But here’s the catch: analyzing and integrating data from multiple tissue slices and finding meaningful patterns within a single slice can be quite challenging. To overcome this hurdle, researchers have developed several algorithms specifically tailored for ST data analysis. These algorithms help identify distinct spatial regions within a tissue slice and align data from different sources for further analysis.
To guide researchers in choosing the right methods and paving the way for future advancements, a team of scientists conducted a comprehensive benchmarking study. They evaluated various state-of-the-art algorithms by analyzing real and simulated datasets with different sizes, technologies, species, and complexities.The researchers assessed each algorithm using a range of quantitative and qualitative metrics. These metrics included measures of clustering accuracy, visualization techniques to understand spatial relationships, alignment accuracy, and even 3D reconstruction. By considering both method performance and data quality, they provided a holistic evaluation to aid researchers in selecting the best tools for their specific needs.
The team has made all their evaluation code available on GitHub, along with online notebooks and documentation. This ensures transparency and reproducibility, allowing other researchers to validate the benchmarking results and explore new methods using different datasets.In conclusion, this groundbreaking study provides comprehensive recommendations to researchers, offering guidance in choosing optimal tools and inspiring future developments. With these advanced techniques, we are unlocking new possibilities and gaining deeper insights into the fascinating world of complex tissues.
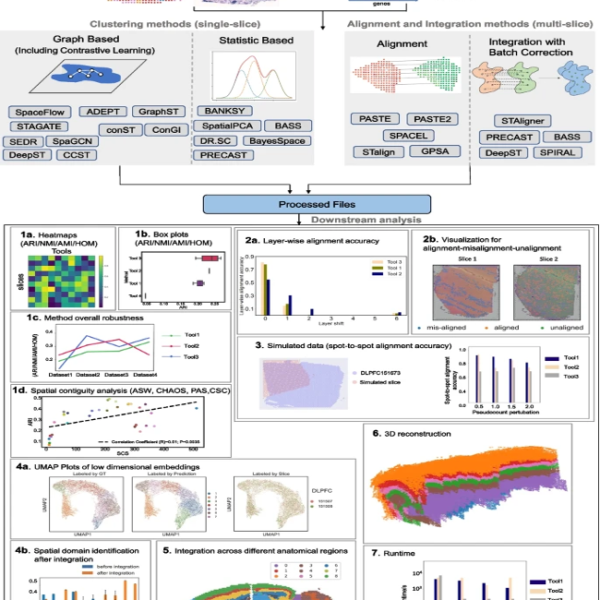