Lening Cui, Christopher Khan, Ying-Chun Pan, Siegfried Schlunk, Emelina Vienneau, Emmanuel Ofosu, Jason Harbert, Ryan LeFevre, Susan Eagle, and Brett Byram. “Using Domain Adaptive Deep Neural Networks to Improve Transthoracic Echocardiography.” Proceedings of SPIE Medical Imaging 2024: Ultrasonic Imaging and Tomography, vol. 12932, 129320M, 2024, San Diego, California
Previous research demonstrated that domain adaptive deep neural networks (DNNs) can surpass delay-and-sum (DAS) beamforming in abdominal imaging. This study explores the potential of applying the domain adaptive DNN framework to transthoracic echocardiography (TTE). Additionally, architectural enhancements such as using an encoder-decoder structure and skip connections are proposed to improve ultrasound image quality for tasks like detecting thrombi in the left atrial appendage (LAA).
The DNN training data comprised both simulated and in vivo cardiac data. Simulated anechoic and hypoechoic cysts with varying levels of clutter were created using Field II, while in vivo data were obtained from patients at Vanderbilt University Medical Center. Fundamental frequency TTE data from five separate cases were processed using DAS, ADMIRE, the baseline model, and several models with modified architectures.
The study found that, regardless of the amount of training data, DNNs consistently achieved higher generalized contrast-to-noise ratio (gCNR) and contrast ratio (CR) but lower contrast-to-noise ratio (CNR) compared to DAS. The best-performing beamformer was a DNN with the proposed architectural improvements, achieving average gCNR and CR values of 0.907 and 48.30 dB, respectively, compared to the baseline DNN values of 0.788 and 39.45 dB, and DAS values of 0.717 and 14.08 dB.
These results indicate that the domain adaptive DNN framework is effective for transthoracic cardiology applications. Moreover, the encoder-decoder architecture with skip connections can further enhance image quality. Future advancements may yield even greater improvements in ultrasound imaging quality.
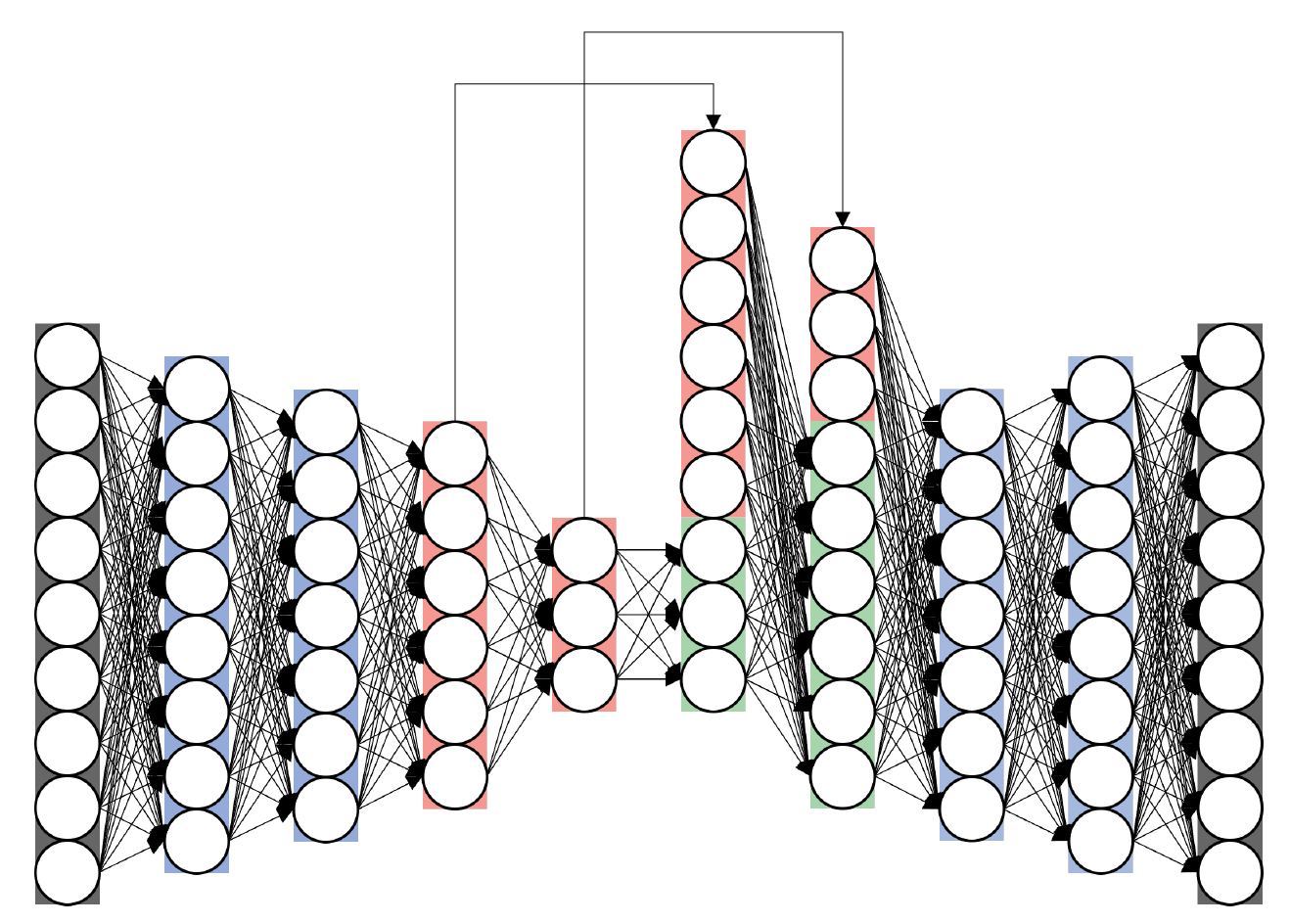
layers; the red layers depict the layers that used skip connections.