Chenyu Gao, Michael E. Kim, Ho Hin Lee, Qi Yang, Nazirah Mohd Khairi, Praitayini Kanakaraj, Nancy R. Newlin, Derek B. Archer, Angela L. Jefferson, Warren D. Taylor, Brian D. Boyd, Lori L. Beason-Held, Susan M. Resnick, Yuankai Huo, Katherine D. Van Schaik, Kurt G. Schilling, Daniel Moyer, Ivana Išgum, and Bennett A. Landman. “Predicting Age from White Matter Diffusivity with Residual Learning.” Proceedings of SPIE Medical Imaging 2024: Image Processing, vol. 12926, 129262I, 2024, San Diego, California
Imaging findings that are inconsistent with expected results for specific chronological age ranges can serve as early indicators of neurological disorders and increased mortality risk. Estimating chronological age from structural MRI data, and identifying deviations from expected results, has become crucial for developing biomarkers sensitive to such deviations. Alongside structural analysis, diffusion tensor imaging (DTI) has shown effectiveness in detecting age-related microstructural changes in brain white matter, making it a promising modality for brain age prediction.
Although early studies have attempted to use DTI for age estimation, it remains unclear whether the success of these predictions is due to the unique microstructural and diffusivity features of DTI or the macrostructural features also present in DTI data. This study aims to develop white-matter-specific age estimation by focusing solely on microstructural features and ignoring macrostructural information when predicting age from DTI scalar images.
Two distinct methods were employed. The first method involved extracting only microstructural features from regions of interest. The second method utilized 3D residual neural networks (ResNets) to learn features directly from images that were non-linearly registered and warped to a template, minimizing macrostructural variations.
When tested on unseen data, the first method yielded a mean absolute error (MAE) of 6.11 years for cognitively normal participants and 6.62 years for cognitively impaired participants. The second method, using ResNets, achieved a MAE of 4.69 years for cognitively normal participants and 4.96 years for cognitively impaired participants. These results suggest that the ResNet model effectively captures subtler, non-macrostructural features for brain age prediction, highlighting its potential for more accurate age estimation from DTI data.
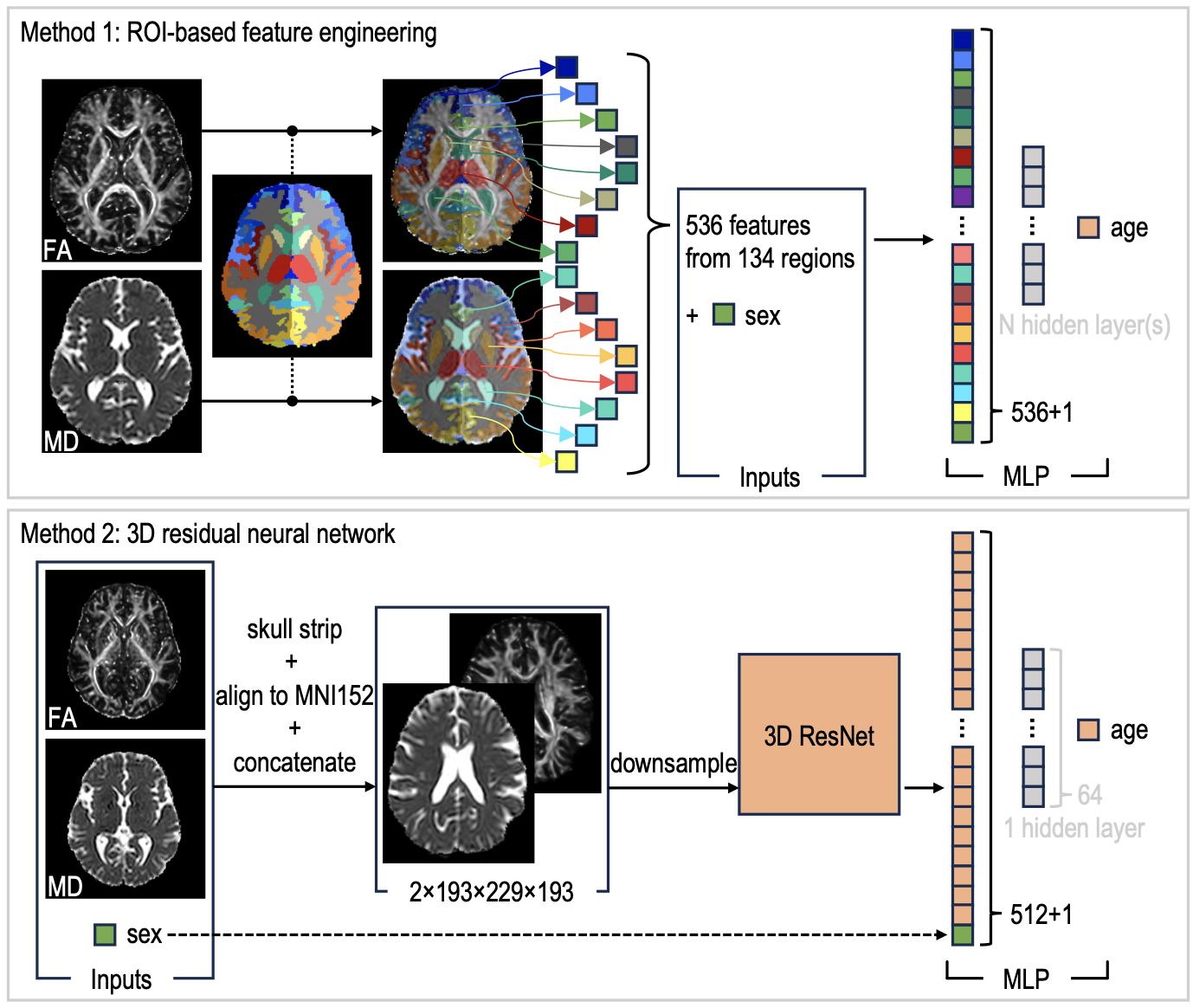