Lucas W. Remedios, Shunxing Bao, Samuel W. Remedios, Ho Hin Lee, Leon Cai, Thomas Li, Ruining Deng, Can Cui, Jia Li, Qi Liu, Ken S. Lau, Joseph T. Roland, Mary K. Washington, Lori A. Coburn, Keith T. Wilson, Yuankai Huo, and Bennett A. Landman. “Nucleus Subtype Classification Using Inter-modality Learning.” Proceedings of SPIE Medical Imaging 2024: Digital and Computational Pathology, vol. 12933, 129330F, 2024, San Diego, California,
Understanding how cells communicate, co-locate, and interrelate is essential for grasping human physiology. Hematoxylin and eosin (H&E) staining is widely used in clinical studies and research. The Colon Nucleus Identification and Classification (CoNIC) Challenge recently advanced artificial intelligence to label six cell types on H&E stained colon tissues. However, this only covers a small fraction of potential cell classifications, missing various epithelial (progenitor, endocrine, goblet), lymphocyte (B cells, helper T cells, cytotoxic T cells), and connective tissue (fibroblasts, stromal) subtypes.
To address this limitation, the study proposes using inter-modality learning to label previously unclassifiable cell types on virtual H&E images. The researchers utilized multiplexed immunofluorescence (MxIF) histology imaging to identify 14 cell type subclasses. By performing style transfer, they synthesized virtual H&E images from MxIF and transferred the detailed labels from MxIF to these virtual H&E images. They then assessed the effectiveness of this learning approach.
The results demonstrated that helper T cells and progenitor cells could be identified with positive predictive values of 0.34±0.15 (prevalence 0.03±0.01) and 0.47±0.1 (prevalence 0.07±0.02) respectively on virtual H&E images. This method represents a promising step toward automating cell type annotation in digital pathology, significantly enhancing the capability to classify diverse cell types beyond current limitations.
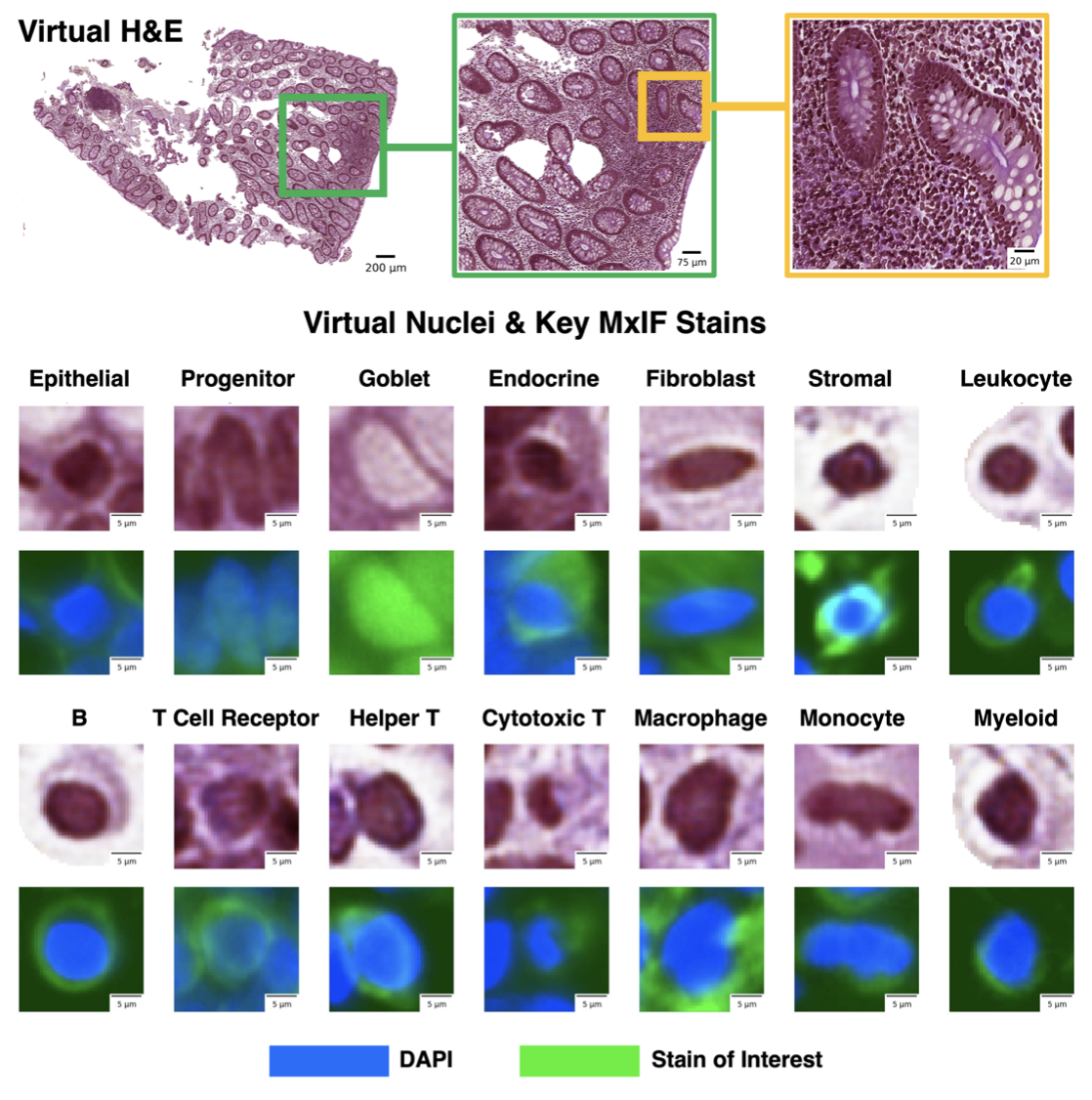