Praitayini Kanakaraj, Tianyuan Yao, Nancy R. Newlin, Leon Y. Cai, Kurt G. Schilling, Baxter P. Rogers, Adam Anderson, Daniel Moyer, and Bennett A. Landman. “Nonlinear Gradient Field Estimation in Diffusion MRI Tensor Simulation.” Proceedings of SPIE Medical Imaging 2024: Physics of Medical Imaging, vol. 12925, 1292549, 2024, San Diego, California
Gradient nonlinearities in magnetic resonance imaging (MRI) not only cause spatial distortions but also create discrepancies between the intended and acquired diffusion sensitization in diffusion-weighted (DW) MRI. With advances in scanner performance, correcting these gradient nonlinearities has become increasingly important. Common methods for estimating gradient nonlinear fields rely on phantom calibration field maps, which are often impractical, especially for retrospective data.
This study presents a new approach to estimate the complete gradient nonlinear field, denoted as L(r), by formulating a quadratic minimization problem. This method begins with the corrupted diffusion signal and estimates L(r) under two scenarios: (1) when the true diffusion tensor is known, and (2) when the true diffusion tensor is unknown and must be estimated. The validity of this mathematical approach is demonstrated both theoretically and through tensor simulation.
The estimated field is evaluated using diffusion tensor metrics: mean diffusivity (MD), fractional anisotropy (FA), and principal eigenvector (V1). Simulations with 300 diffusion tensors indicate that the formulation is stable and not ill-posed. When the true diffusion tensor is known, the change in the determinant of the estimated L(r) field relative to the true field is near zero, and the median difference in corrected diffusion metrics compared to true values is also near zero. The results show that the accuracy of L(r) estimation depends on the level of corruption in L(r).
This work introduces a novel mathematical method to estimate the gradient field without requiring additional calibration scans, offering a significant advancement for correcting gradient nonlinearities in DW MRI.
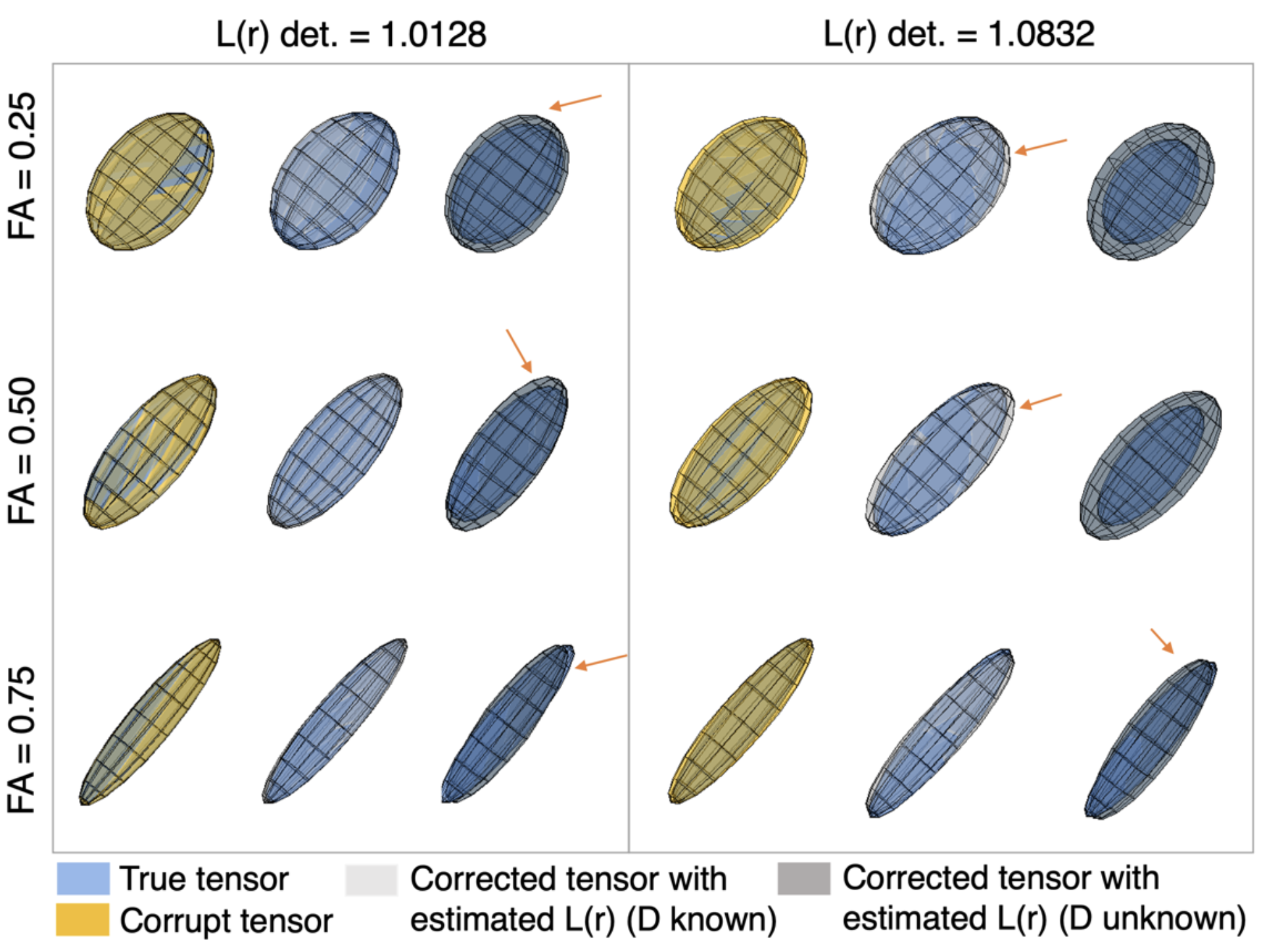
tensors are shown for FA values 0.25, 0.50, and 0.75 when SNR = 30. Corrected diffusion tensor overlaid with true
tensor (column 2 and 3) appear alike when determinant = 1.0128, while with determinant = 1.0832 there are slight
variations between the estimated and true tensors (column 5 and 6).