Franklin Hu, Ruining Deng, Shunxing Bao, Haichun Yang, and Yuankai Huo. “Multi-scale Multi-site Renal Microvascular Structures Segmentation for Whole Slide Imaging in Renal Pathology.” Proceedings of SPIE Medical Imaging 2024: Digital and Computational Pathology, vol. 12933, 1293319, 2024, San Diego, California, United States.
Segmenting tiny blood vessels, like arterioles, venules, and capillaries, in kidney tissue images is crucial for kidney disease research but is currently very time-consuming when done manually. To automate this process, researchers developed Omni-Seg, a new method that uses deep learning to handle data from various sources and scales. Unlike most existing methods that are limited to specific data sets, Omni-Seg can learn from images that are only partially labeled, where each image has only one type of tissue labeled.
The researchers trained Omni-Seg on images from two different databases, HuBMAP and NEPTUNE, using multiple magnification levels (40x, 20x, 10x, and 5x). The results showed that Omni-Seg performed better in accurately identifying and segmenting these blood vessels, achieving higher scores on measures of accuracy, the Dice Similarity Coefficient (DSC), and Intersection over Union (IoU). This method offers a powerful tool for renal pathologists, making it easier and faster to analyze kidney tissue images quantitatively.
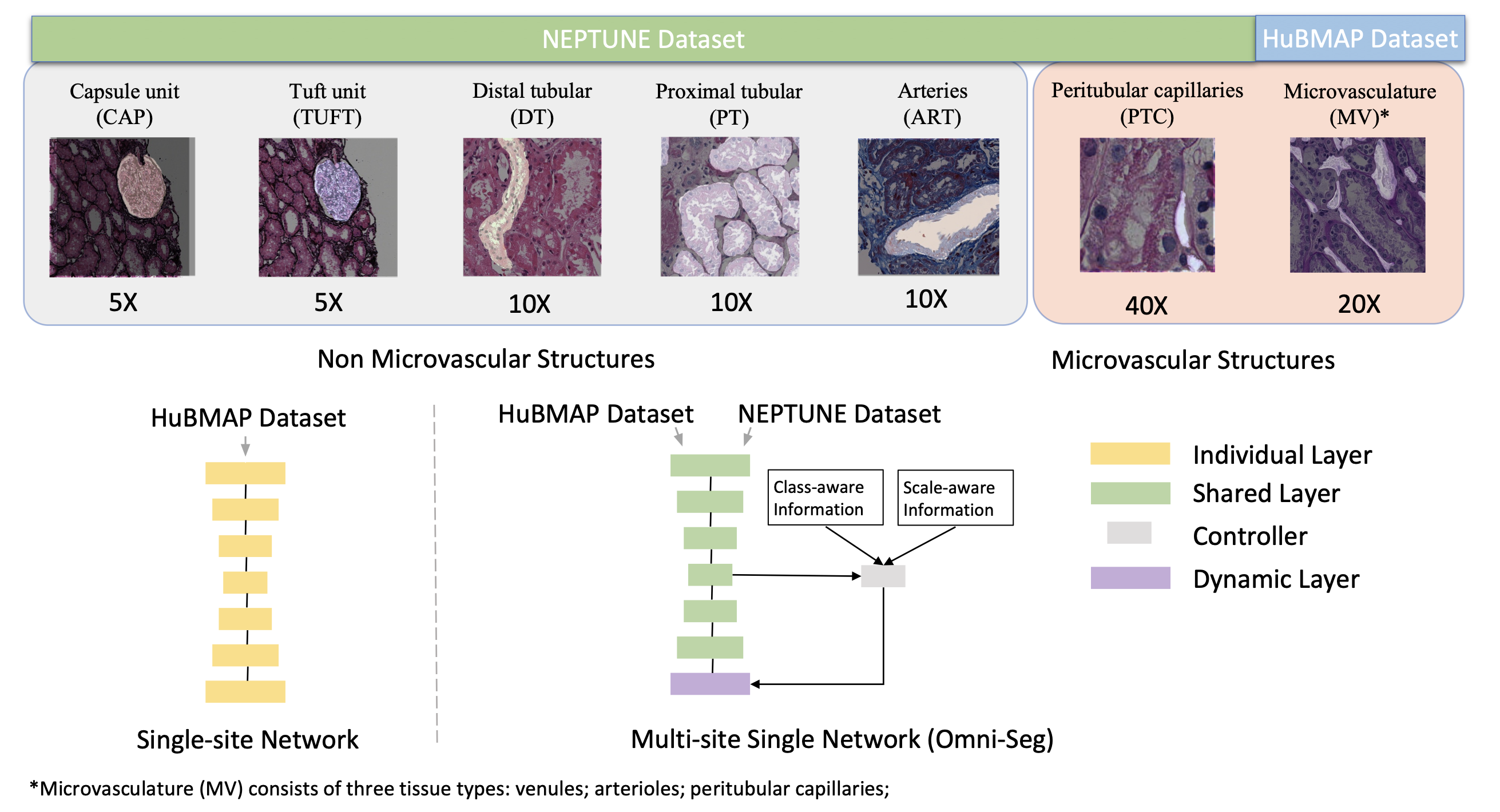