Hanyu Zheng, Quan Liu, You Zhou, Ivan I. Kravchenko, Yuankai Huo, and Jason Valentine. “Meta-optic Accelerators for Object Classifiers.” Science Advances, vol. 8, no. 30, 27 July 2022.
Rapid advances in deep learning have led to significant transformations across various fields, including medical image analysis and autonomous systems. These advances, however, have resulted in digital neural networks with substantial computational requirements, leading to high energy consumption and challenges in real-time decision-making, particularly when computational resources are limited.
This study presents a meta-optic based neural network accelerator designed to offload computationally intensive convolution operations to high-speed, low-power optics. The architecture utilizes metasurfaces to enable spatial multiplexing and incorporate additional information channels, such as polarization, for object classification. An end-to-end design approach co-optimizes both the optical and digital systems, resulting in a robust classifier.
The classifier demonstrates a 95% accuracy in classifying handwritten digits and 94% accuracy in classifying both the digit and its polarization state. This innovative approach has the potential to enable compact, high-speed, and low-power image and information processing systems, with broad applications in machine vision and artificial intelligence.
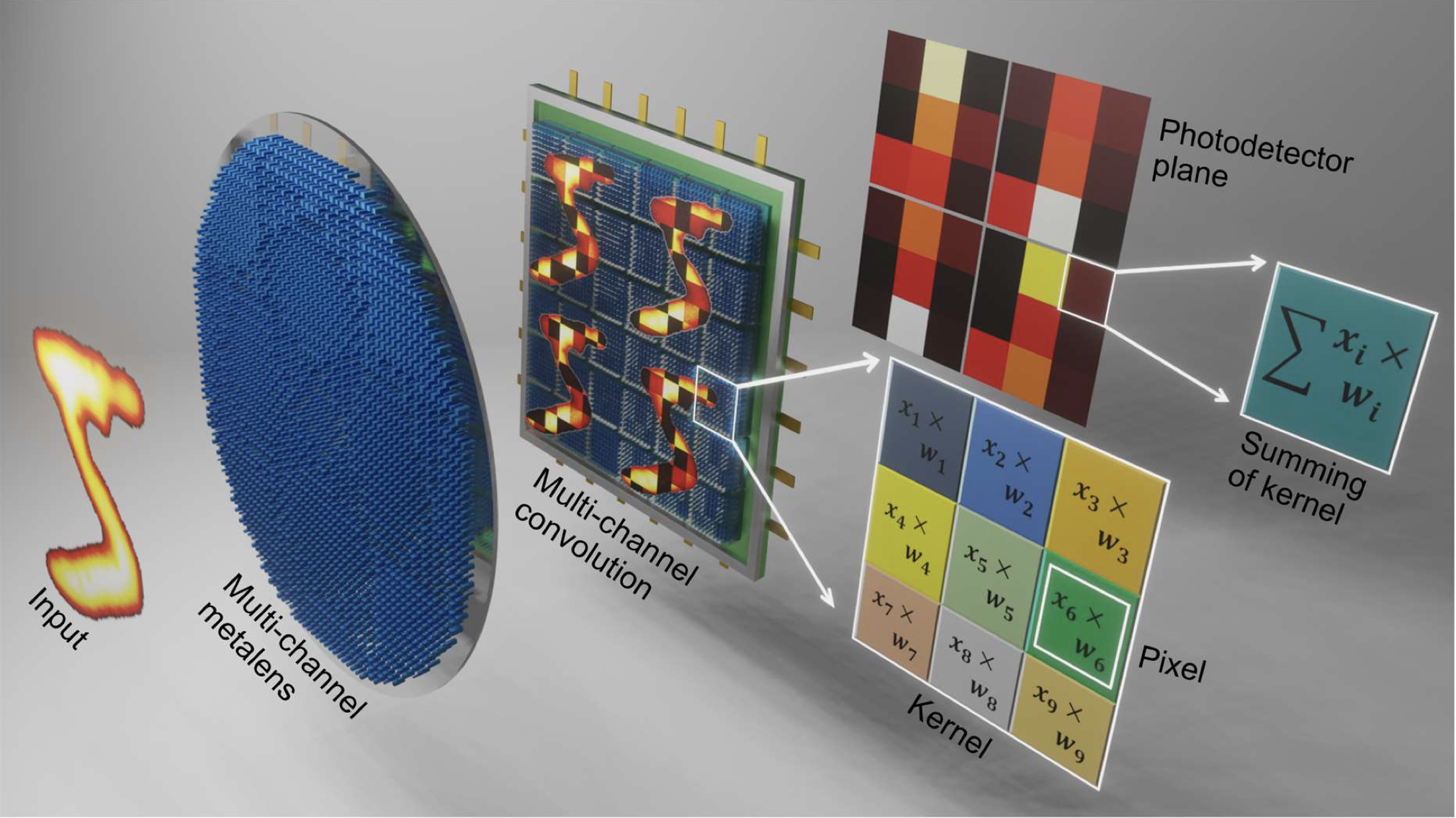