Yamin Li, Ange Lou, Ziyuan Xu, Shiyu Wang, and Catie Chang. “Leveraging Sinusoidal Representation Networks to Predict fMRI Signals from EEG.” Proceedings of SPIE Medical Imaging 2024: Image Processing, vol. 12926, 129263A, 2024, San Diego, California
Functional magnetic resonance imaging (fMRI) is a vital tool in neuroscience for observing brain activity, but it has limitations such as hemodynamic blurring, high cost, immobility, and incompatibility with metal implants. Electroencephalography (EEG), while offering high temporal resolution by directly recording cortical electrical activity, has limited spatial resolution and cannot capture data from deep brain structures. Combining the strengths of both methods by deriving fMRI information from EEG could provide a cost-effective way to image a broader range of brain regions and enhance the interpretation of fMRI signals.
To address the challenge of modeling fMRI from EEG, given the high dimensionality and artifact-prone nature of both modalities, a novel architecture is proposed. This model predicts fMRI signals directly from multi-channel EEG without the need for explicit feature engineering. It employs a Sinusoidal Representation Network (SIREN) to learn frequency information in brain dynamics from EEG data, which is then input to an encoder-decoder system to reconstruct the fMRI signal for specific brain regions.
The model was tested using a simultaneous EEG-fMRI dataset from eight subjects, focusing on its ability to predict subcortical fMRI signals. Results show that this new model outperforms recent state-of-the-art models, demonstrating the potential of using periodic activation functions in deep neural networks for functional neuroimaging data. This approach could significantly expand the capabilities of EEG and improve the cost-effectiveness and accessibility of brain imaging.
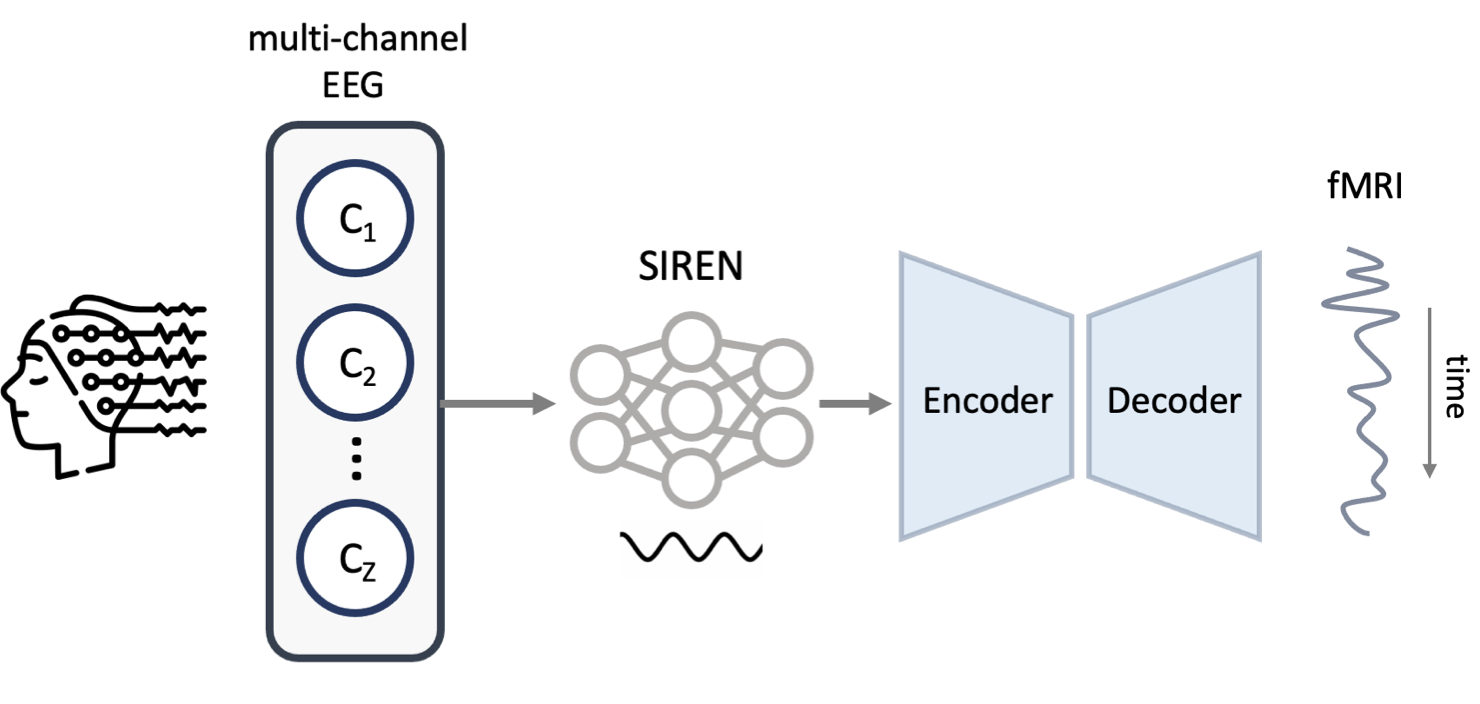