Xueyuan Li, Ruining Deng, Yucheng Tang, Shunxing Bao, Haichun Yang, and Yuankai Huo. “Leverage Weekly Annotation to Pixel-wise Annotation via Zero-shot Segment Anything Model for Molecular-empowered Learning.” Proceedings of SPIE Medical Imaging 2024: Digital and Computational Pathology, vol. 12933, 129330K, 2024, San Diego, California
Precise identification of multiple cell classes in high-resolution Giga-pixel whole slide imaging (WSI) is essential for various clinical applications. Building an AI model for this purpose usually requires pixel-level annotations, which are time-consuming, require domain expertise (e.g., pathologists), and are prone to errors, particularly when differentiating intricate cell types (e.g., podocytes and mesangial cells) through visual inspection alone.
A recent study found that lay annotators could sometimes outperform domain experts in labeling tasks when provided with additional immunofluorescence (IF) images for reference, a method termed molecular-empowered learning. However, the manual delineation required for these annotations remains a resource-intensive task.
This paper explores bypassing pixel-level delineation by using the recent segment anything model (SAM) with weak box annotations in a zero-shot learning approach. SAM’s capability to generate pixel-level annotations from box annotations is leveraged to train a segmentation model. The findings indicate that SAM-assisted molecular-empowered learning (SAM-L) reduces the annotation effort required from lay annotators by necessitating only weak box annotations, without compromising annotation accuracy or the performance of the deep learning-based segmentation.
This research represents a significant advancement in making the annotation process for training pathological image segmentation more accessible, relying solely on non-expert annotators.
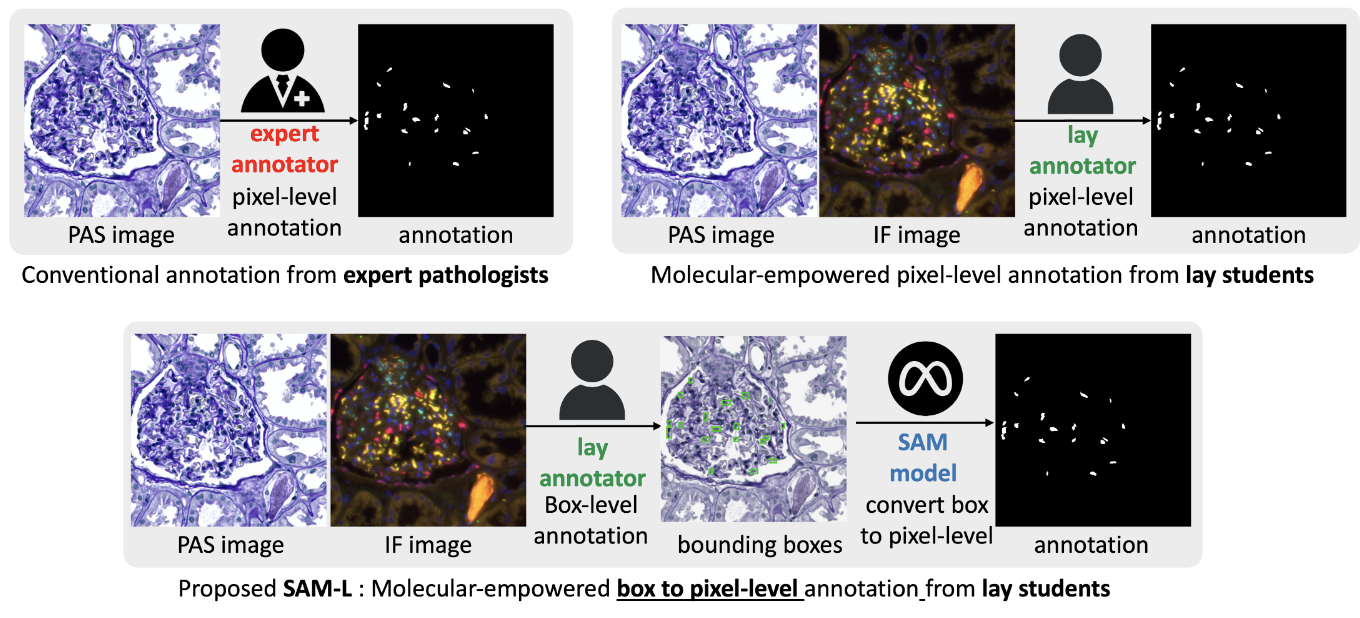