Tianyuan Yao, Derek B. Archer, Praitayini Kanakaraj, Nancy Newlin, Shunxing Bao, Daniel Moyer, Kurt Schilling, Bennett A. Landman, and Yuankai Huo. “Learning-based Free-water Correction Using Single-shell Diffusion MRI.” Proceedings of SPIE Medical Imaging 2024: Image Processing, vol. 12926, 1292607, 2024, San Diego, California
Diffusion magnetic resonance imaging (dMRI) enables the assessment of subvoxel brain microstructure by extracting biomarkers like fractional anisotropy and reconstructing white matter fiber trajectories to reveal brain connectivity. However, accurate analysis is challenging at the interface between cerebrospinal fluid (CSF) and white matter, where the MRI signal is a mix of contributions from both CSF and white matter partial volumes. This mixture introduces significant bias in estimating diffusion properties, thus limiting the clinical utility of diffusion-weighted imaging (DWI).
Furthermore, existing mathematical models often struggle with single-shell acquisitions, which are common in clinical settings. Without proper regularization, direct model fitting is impractical.
To address these challenges, we propose a novel voxel-based deep learning method for mapping and correcting free-water partial volume contamination in DWI. This approach leverages data-driven techniques to reliably estimate plausible free-water volumes across various diffusion MRI acquisition schemes, including single-shell acquisitions. Our evaluation shows that this methodology consistently produces more accurate and reliable results than previous methods.
By effectively mitigating the impact of free water partial volume effects, our approach enhances the accuracy and reliability of DWI analysis for single-shell dMRI. This advancement expands the potential applications of dMRI in assessing brain microstructure and connectivity, making it more useful in clinical settings.
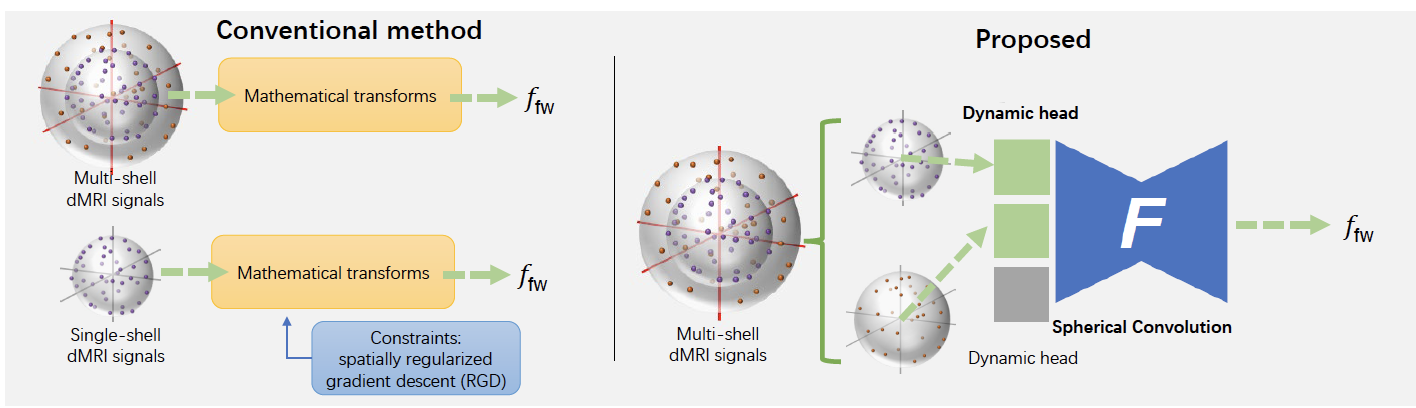
manifold to get a unique solution for the free water elimination on single-shell dMRI. Previous deep learning approaches
achieve accurate model fitting for multi-shell and single-shell data. However, this framework did not allow for variations
in input data size and therefore did not achieve a unified model for both data types. The prediction result shall have a
significant bias when fed with dMRI from an unseen acquisition scheme. In our study, we proposed a single holistic model
for different shell configurations that can recover/predict microstructural measures. Both single-shell and multi-shell dMRI
sequences can be fed into the model together to improve the model performance on various shell configurations.