Aravind R. Krishnan, Kaiwen Xu, Thomas Li, Chenyu Gao, Lucas W. Remedios, Praitayini Kanakaraj, Ho Hin Lee, Shunxing Bao, Kim L. Sandler, Fabien Maldonado, Ivana Išgum, and Bennett A. Landman. “Inter-vendor Harmonization of CT Reconstruction Kernels Using Unpaired Image Translation.” Proceedings of SPIE Medical Imaging 2024: Image Processing, vol. 12926, 129261D, 2024, San Diego, California
The reconstruction kernel in computed tomography (CT) generation determines the image texture, and consistency in reconstruction kernels is crucial because the underlying CT texture can affect quantitative image analysis measurements. Harmonization, or kernel conversion, aims to minimize measurement differences caused by inconsistent reconstruction kernels. Existing methods for CT scan harmonization across single or multiple manufacturers require paired scans of hard and soft reconstruction kernels that are spatially and anatomically aligned, necessitating the training of numerous models across different kernel pairs within manufacturers.
In this study, an unpaired image translation approach was adopted to investigate harmonization between and across reconstruction kernels from different manufacturers. A multipath cycle generative adversarial network (GAN) was constructed, utilizing hard and soft reconstruction kernels from Siemens and GE vendors, sourced from the National Lung Screening Trial dataset. Fifty scans from each reconstruction kernel were used to train the multipath cycle GAN. To evaluate the effect of harmonization on the reconstruction kernels, 50 scans each from Siemens hard kernel, GE soft kernel, and GE hard kernel were harmonized to a reference Siemens soft kernel (B30f), and the percent emphysema was assessed.
A linear model was fitted considering age, smoking status, sex, and vendor, followed by an analysis of variance (ANOVA) on the emphysema scores. The approach minimized differences in emphysema measurement and highlighted the impact of age, sex, smoking status, and vendor on emphysema quantification. This study demonstrates the effectiveness of using unpaired image translation with multipath cycle GANs for kernel harmonization across different manufacturers, improving the consistency and reliability of quantitative image analysis.
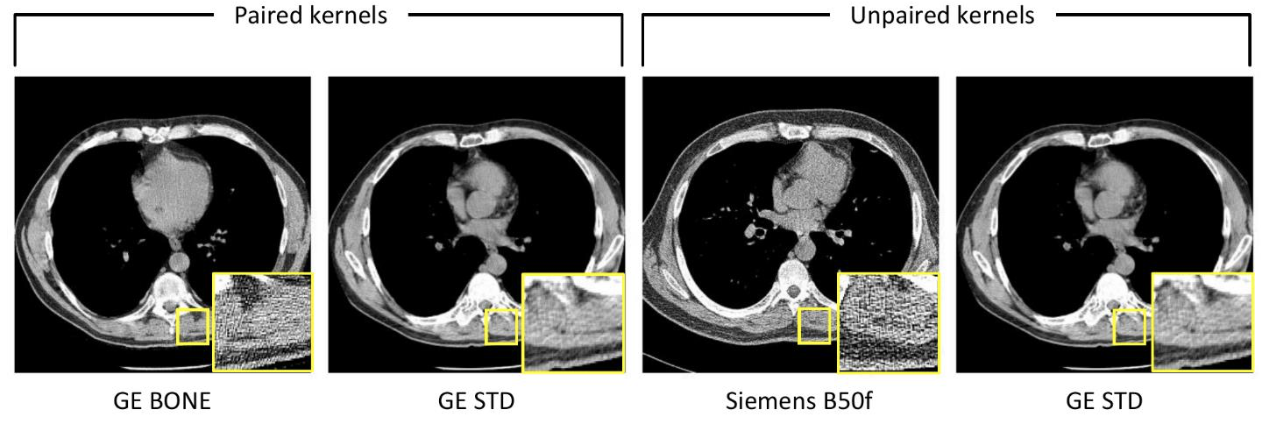