Hanliang Xu, Nancy R. Newlin, Michael E. Kim, Chenyu Gao, Praitayini Kanakaraj, Aravind R. Krishnan, Lucas W. Remedios, Nazirah Mohd Khairi, Kimberly Pechman, Derek Archer, Timothy J. Hohman, Angela L. Jefferson, Ivana Išgum, Yuankai Huo, Daniel Moyer, Kurt G. Schilling, and Bennett A. Landman. “Evaluation of Mean Shift, ComBat, and CycleGAN for Harmonizing Brain Connectivity Matrices Across Sites.” Proceedings of SPIE Medical Imaging 2024: Image Processing, vol. 12926, 129261X, 2024, San Diego, California
Connectivity matrices derived from diffusion MRI (dMRI) offer an interpretable and generalizable way to understand the human brain connectome. However, dMRI is subject to inter-site and between-scanner variations, which can hinder cross-dataset analysis and affect the robustness and reproducibility of results. To evaluate different harmonization approaches on connectivity matrices, we compared graph measures derived from these matrices before and after applying three harmonization techniques: mean shift, ComBat, and CycleGAN.
The sample consisted of 168 age-matched, sex-matched normal subjects from two studies: the Vanderbilt Memory and Aging Project (VMAP) and the Biomarkers of Cognitive Decline Among Normal Individuals (BIOCARD). First, we plotted the graph measures and used the coefficient of variation (CoV) and the Mann-Whitney U test to assess the effectiveness of each method in removing site effects on the matrices and the derived graph measures.
ComBat effectively eliminated site effects for global efficiency and modularity, outperforming the other two methods. However, all methods exhibited poor performance when harmonizing average betweenness centrality. Second, we examined whether the harmonization methods preserved correlations between age and graph measures. All methods, except for CycleGAN in one direction, improved the correlations between age and global efficiency and between age and modularity, changing them from insignificant to significant with p-values less than 0.05.
These findings suggest that while ComBat is particularly effective for certain graph measures, challenges remain in harmonizing other measures like betweenness centrality. Nonetheless, the ability of these methods to enhance the significance of age-related correlations highlights their potential in improving the robustness of dMRI connectivity analyses across different datasets.
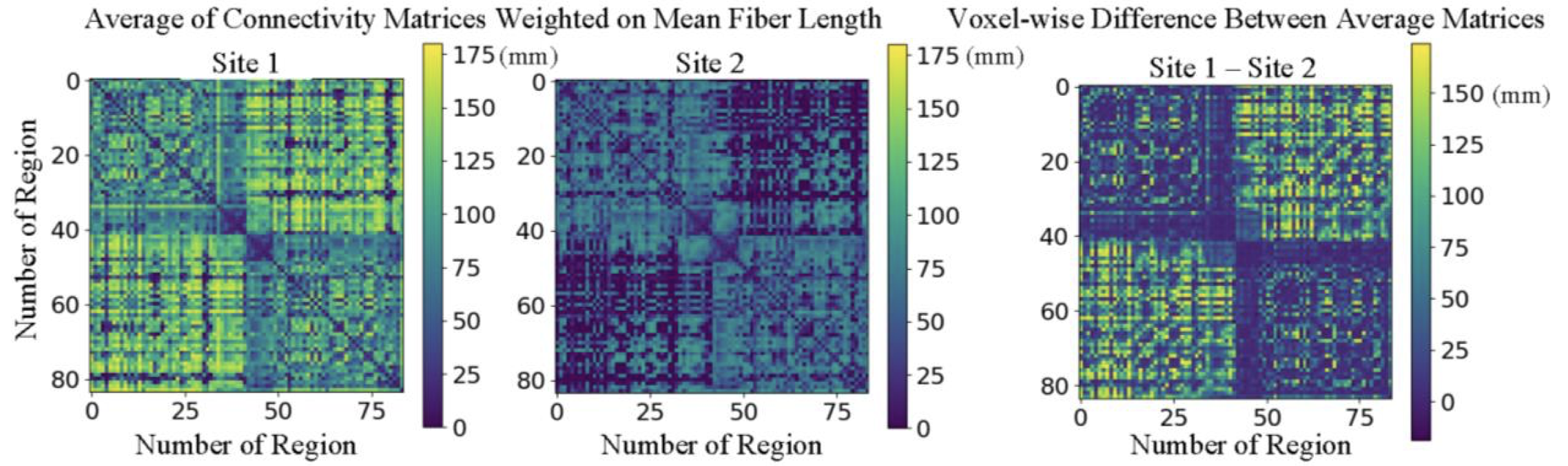
generates tractograms with generally longer streamlines. Note the substantial differences in the first and third quadrant.
Site 2 has fewer, shorter inter-hemispheric streamlines; site 1 has more longer streamlines across hemispheres.