Juming Xiong, Yilin Liu, Ruining Deng, Regina N. Tyree, Hernan Correa, Girish Hiremath, Yaohong Wang, and Yuankai Huo. “Deep Learning-based Open Source Toolkit for Eosinophil Detection in Pediatric Eosinophilic Esophagitis.” Proceedings of SPIE Medical Imaging 2024: Digital and Computational Pathology, vol. 12933, 129330X, 2024, San Diego, California
Eosinophilic Esophagitis (EoE) is a chronic, immune/antigen-mediated esophageal disease characterized by symptoms related to esophageal dysfunction and histological evidence of eosinophil-dominant inflammation. Due to the complex microscopic representation of EoE in imaging, current manual identification methods are labor-intensive and prone to inaccuracies.
This study introduces an open-source toolkit, named Open-EoE, designed for end-to-end whole slide image (WSI) level eosinophil (Eos) detection with a single line of command via Docker. The toolkit supports three state-of-the-art deep learning-based object detection models and optimizes performance through an ensemble learning strategy, enhancing precision and reliability.
Experimental results demonstrate that Open-EoE can efficiently detect Eos on a testing set of 289 WSIs. At the widely accepted diagnostic threshold of ≥15 Eos per high power field (HPF) for EoE, Open-EoE achieved an accuracy of 91%, showing good consistency with pathologist evaluations. This suggests a promising avenue for integrating machine learning methodologies into the diagnostic process for EoE.
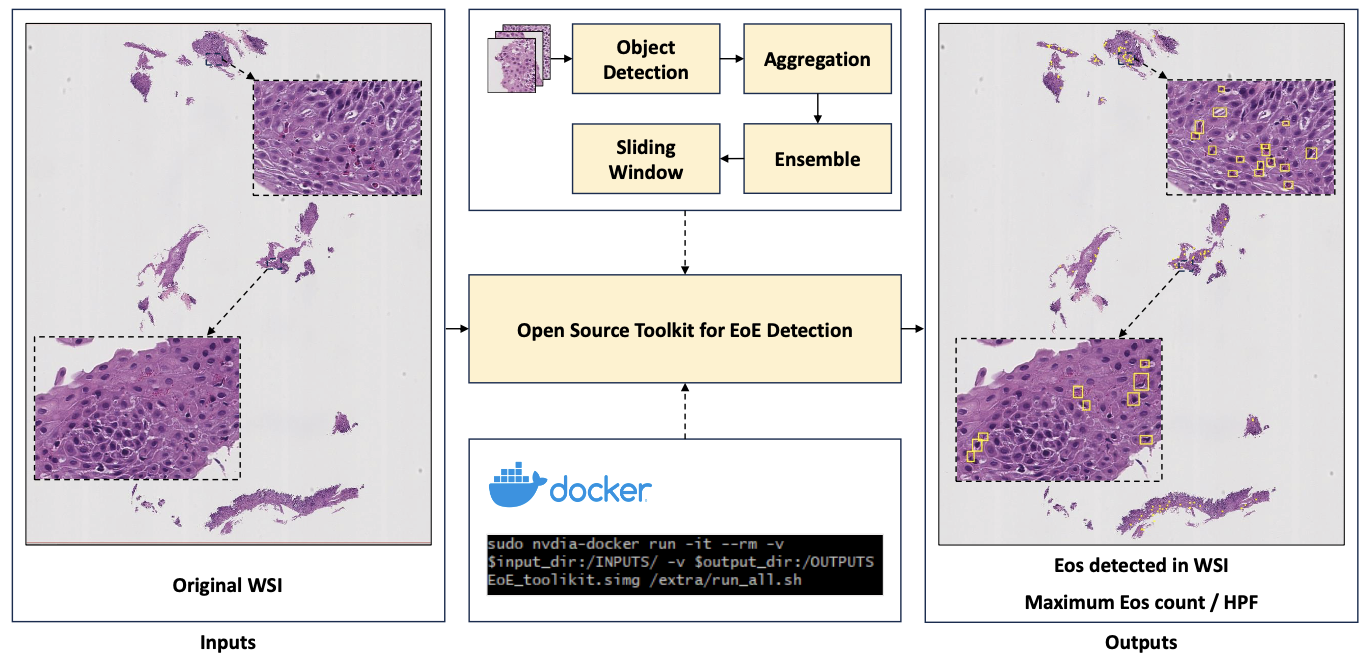