Xin Yu, Qi Yang, Yucheng Tang, Riqiang Gao, Shunxing Bao, Leon Y. Cai, Ho Hin Lee, Yuankai Huo, Ann Zenobia Moore, Luigi Ferrucci, and Bennett A. Landman. “Deep Conditional Generative Model for Longitudinal Single-slice Abdominal Computed Tomography Harmonization.” Journal of Medical Imaging (Bellingham), vol. 11, no. 2, 024008, March 2024. doi:10.1117/1.JMI.11.2.024008.
Two-dimensional single-slice abdominal computed tomography (CT) provides detailed, high-resolution tissue maps that allow for the quantitative characterization of relationships between health conditions and aging. However, longitudinal analysis of body composition changes using these scans is challenging due to positional variation between slices acquired at different times, leading to the capture of different organs and tissues.
To address this issue, we propose C-SliceGen, a method that takes an arbitrary axial slice in the abdominal region as a condition and generates a predefined vertebral level slice by estimating structural changes in the latent space. Our experiments on 2,608 volumetric CT scans from two in-house datasets and 50 subjects from the 2015 Multi-Atlas Abdomen Labeling Challenge Beyond the Cranial Vault (BTCV) dataset demonstrate that our model can generate high-quality, realistic images that are similar to the target slices.
We further evaluated our method’s ability to harmonize longitudinal positional variation on 1,033 subjects from the Baltimore Longitudinal Study of Aging dataset, which contains longitudinal single abdominal slices. The results confirmed that our method can harmonize slice positional variance, particularly in terms of visceral fat area.
This approach offers a promising direction for mapping slices from different vertebral levels to a target slice, reducing positional variance for single-slice longitudinal analysis. The source code for C-SliceGen is available at: https://github.com/MASILab/C-SliceGen.
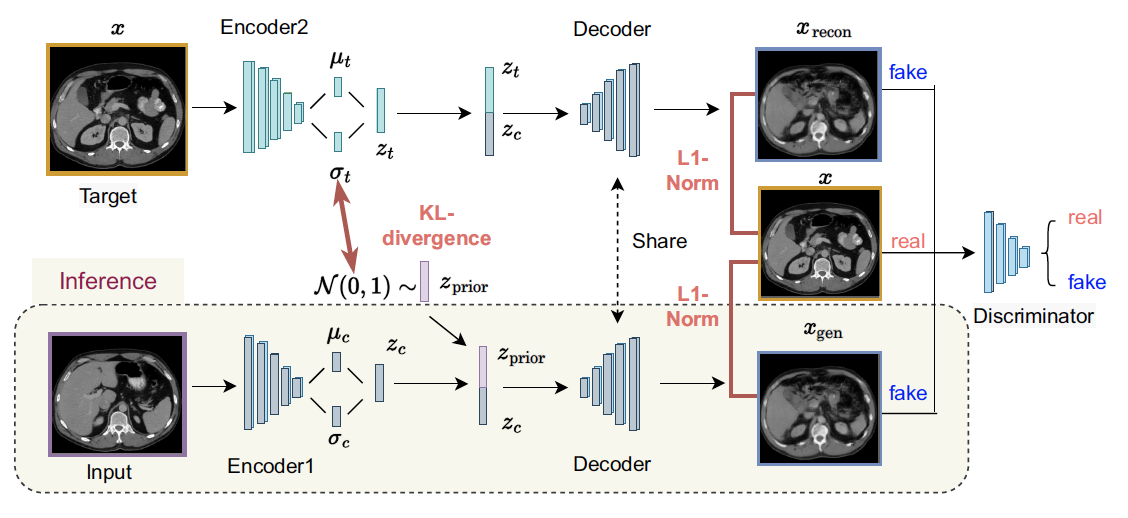
phase, target images (x) are used as the GT for the generation and reconstruction process. Latent
variables, such as zc , zt , and zprior, are derived from conditional images, target images, and
the normal Gaussian distribution, respectively. xgen and xrecon are considered fake images and
target images are considered real images for the discriminator.