Reinke, A., Tizabi, M. D., Baumgartner, M., Eisenmann, M., Heckmann-Nötzel, D., Kavur, A. E., Rädsch, T., Sudre, C. H., Acion, L., Antonelli, M., Arbel, T., Bakas, S., Benis, A., Buettner, F., Cardoso, M. J., Cheplygina, V., Chen, J., Christodoulou, E., Cimini, B. A., … Maier-Hein, L. (2024). Understanding metric-related pitfalls in image analysis validation. Nature Methods, 21(2), 182–194. https://doi.org/10.1038/S41592-023-02150-0
A recent study tackles the issue of choosing the right validation metrics in image analysis, crucial for both advancing scientific research and applying findings in practical settings. The researchers used a multistage Delphi process involving experts from various disciplines, coupled with extensive community feedback, to gather and synthesize information about common pitfalls in selecting validation metrics. This effort led to the creation of a comprehensive, easily accessible resource that categorizes these pitfalls using a new, universally applicable taxonomy. While this work specifically addresses biomedical image analysis, the insights it provides are applicable across various fields, aiming to improve understanding and decision-making regarding validation metrics globally. This is a significant step towards closing the gap between artificial intelligence research and real-world application.
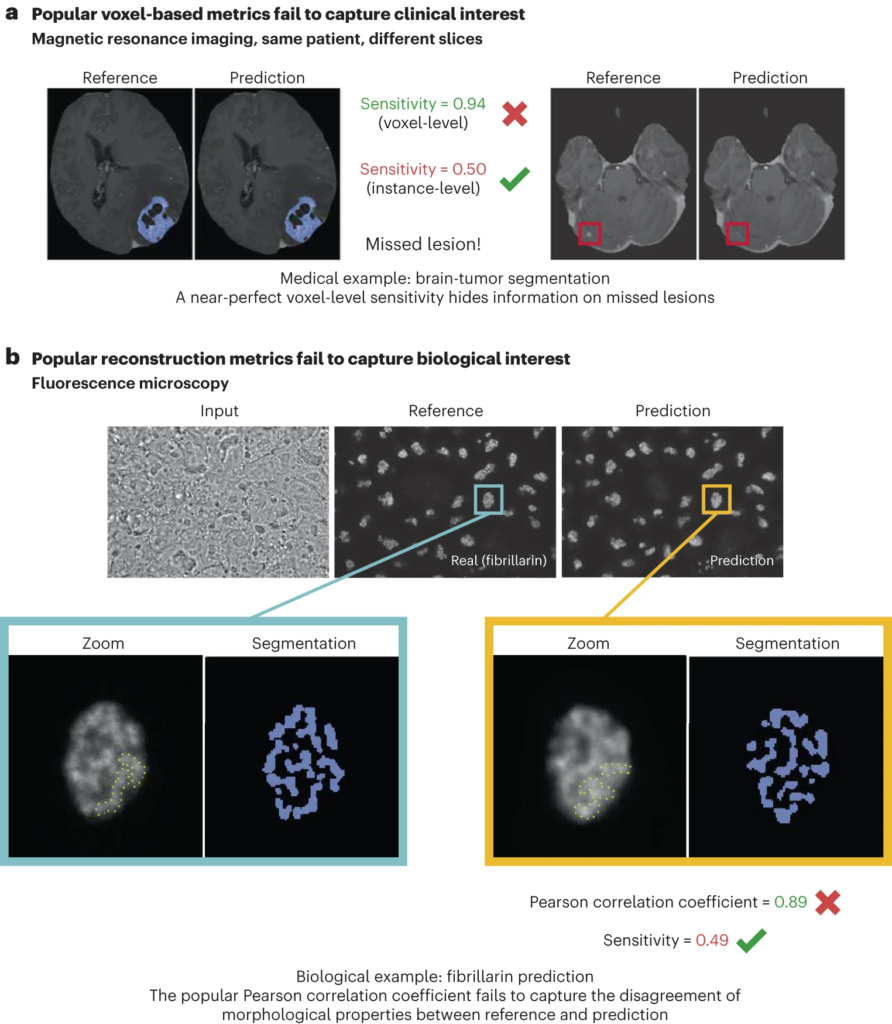