Maier-Hein, L., Reinke, A., Godau, P., Tizabi, M. D., Buettner, F., Christodoulou, E., Glocker, B., Isensee, F., Kleesiek, J., Kozubek, M., Reyes, M., Riegler, M. A., Wiesenfarth, M., Kavur, A. E., Sudre, C. H., Baumgartner, M., Eisenmann, M., Heckmann-Nötzel, D., Rädsch, T., … Jäger, P. F. (2024). Metrics reloaded: recommendations for image analysis validation. Nature Methods, 21(2), 195–212. https://doi.org/10.1038/S41592-023-02151-Z
The study introduces “Metrics Reloaded,” a comprehensive framework designed to assist researchers in selecting the most appropriate validation metrics for machine learning (ML) algorithms, particularly in biomedical image analysis. This framework addresses a common issue where the performance metrics often fail to reflect the actual domain interests, thereby impeding scientific progress and the practical application of ML techniques. “Metrics Reloaded” was developed by an international consortium through a multistage Delphi process and introduces a novel concept known as a “problem fingerprint.” This is a structured representation that encapsulates all relevant aspects for metric selection, including domain interest, characteristics of the target structures, dataset, and algorithm outputs. The framework guides users in choosing and applying suitable validation metrics, alerting them to potential pitfalls. It supports various image analysis tasks like image-level classification, object detection, and segmentation. To enhance accessibility and usability, the framework has been implemented in an online tool, “Metrics Reloaded,” promoting the standardization of validation methods across different application domains and demonstrating its utility through several biomedical case studies.
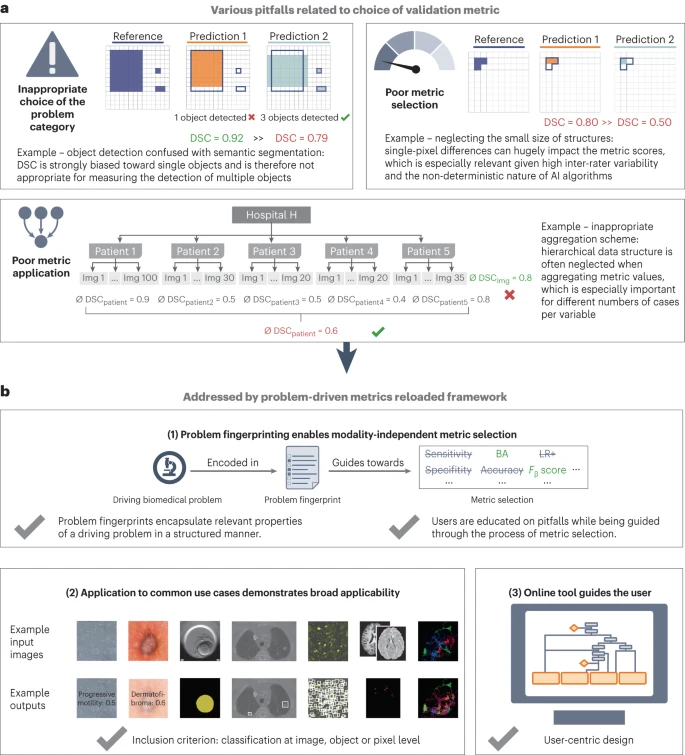