Krishnan, A. R., Xu, K., Li, T. Z., Remedios, L. W., Sandler, K. L., Maldonado, F., & Landman, B. A. (2024). Lung CT harmonization of paired reconstruction kernel images using generative adversarial networks. Medical Physics. https://doi.org/10.1002/MP.17028
In a study focused on the harmonization of reconstruction kernels in CT imaging, researchers utilized the National Lung Screening Trial to examine the impact of kernel choice on CT image texture and quantitative assessments. The study involved comparing CT scans reconstructed with both soft and hard tissue kernels, using a deep learning model based on the pix2pix architecture to convert images between these kernel types. A total of 1000 paired scans were used, with each model trained and tested on 100 pairs. The effectiveness of the conversion was measured through image similarity metrics such as root mean squared error (RMSE), peak signal-to-noise ratio (PSNR), and structural similarity index measure (SSIM). The results showed significant improvements in these metrics post-conversion, indicating successful kernel harmonization. This conversion also led to more consistent measurements of emphysema, muscle area, and subcutaneous adipose tissue, reducing the variability caused by different kernel types. Additionally, radiomic features remained reproducible after harmonization, underscoring the potential of this deep learning approach to enhance the accuracy and reliability of quantitative CT-based assessments across different imaging settings.
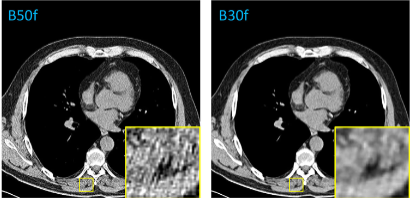