Zheng H, Liu Q, Kravchenko II, Zhang X, Huo Y, Valentine JG. Multichannel meta-imagers for accelerating machine vision. Nat Nanotechnol. 2024 Jan 4. doi: 10.1038/s41565-023-01557-2. Epub ahead of print. PMID: 38177276.
The study introduces a novel “meta-imager” that combines high-speed, low-power optical components with a digital backend to enhance machine vision systems, reducing the heavy computational load typically associated with digital neural networks. This innovative device utilizes metasurfaces for angle and polarization multiplexing, allowing it to perform complex convolution operations—essential for tasks like object classification—in a single optical shot. This integration effectively offloads much of the computational burden from the digital components to the optics, greatly reducing energy consumption and improving processing speed. The meta-imager demonstrated impressive performance, with 98.6% accuracy in classifying handwritten digits and 88.8% accuracy with fashion images. Given its compactness, efficiency, and speed, this technology shows great potential for a broad range of applications in artificial intelligence and machine vision fields, particularly in environments where real-time decision-making is crucial and computational resources are limited.
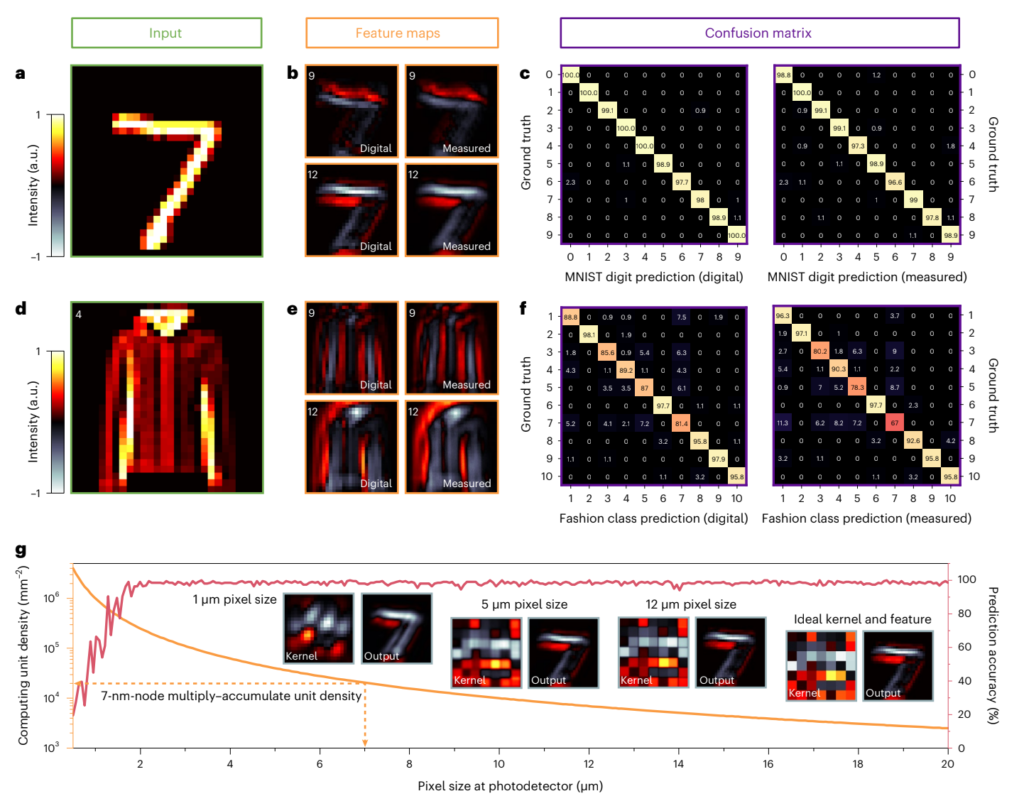
Classification of MNIST and Fashion MNIST objects. a, An input image from the MNIST dataset. b, Ideal and experimentally measured feature maps corresponding to the convolution of the data in a with channels 9 and 12. The top-left corner label indicates the channel number during convolution. c, Comparison between the theoretical and measured confusion matrices for MNIST classification. d, An input image from the Fashion MNIST dataset. The top-left corner label indicates the object class number. e, Ideal and experimentally measured feature maps corresponding to the convolution of the data in d with channels 9 and 12. The top-left corner label indicates the channel number during convolution. f, Comparison between the theoretical and measured confusion matrices for Fashion MNIST classification. g, Predicted accuracy curve for the MNIST dataset and the areal density of the basic computing unit as a function of pixel size. The insets depict the kernel profiles and feature maps at different pixel sizes.